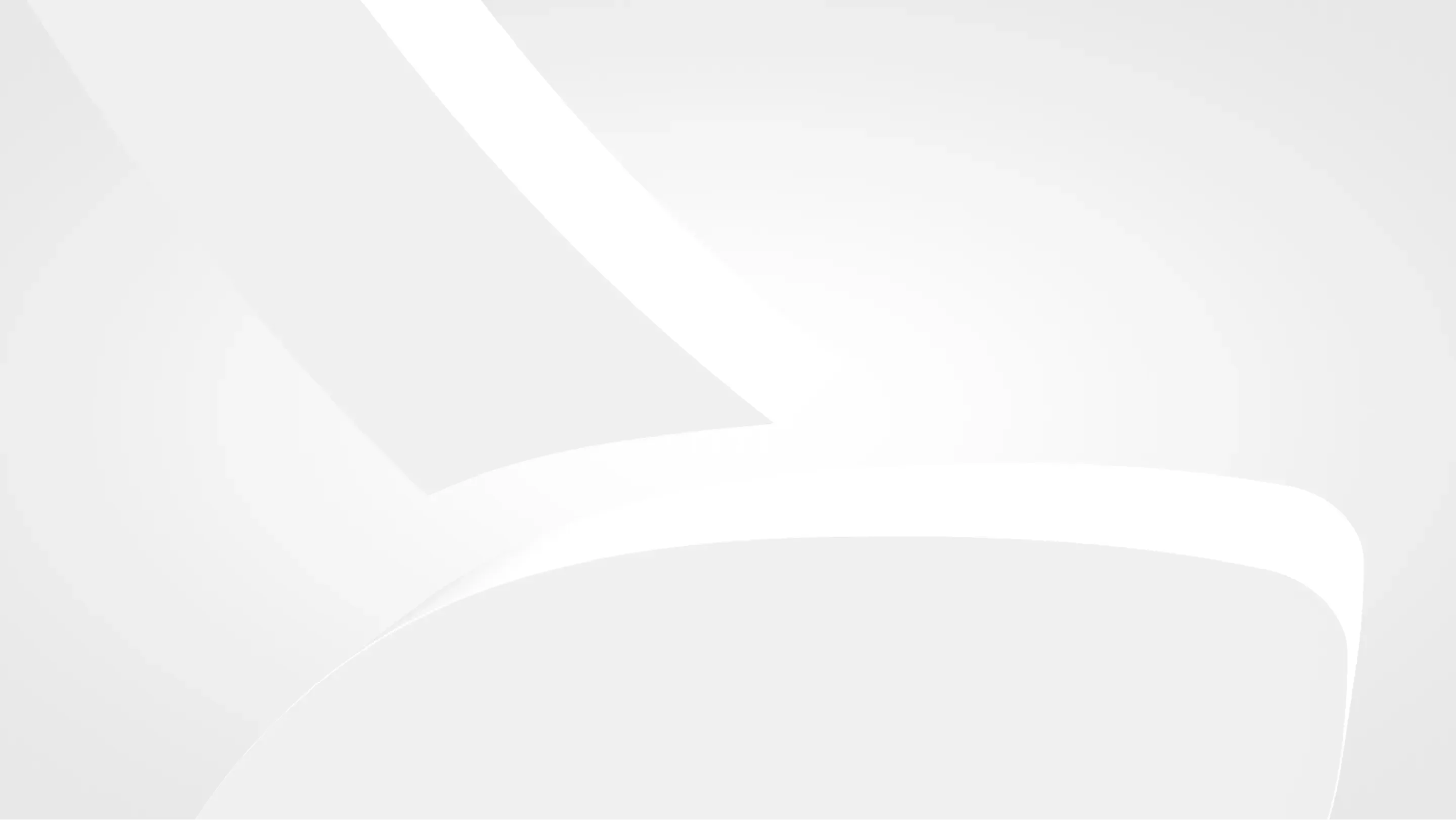
Fine-tune for Reliable and Accurate AI Model Outputs
Fine-Tune AI Models to Improve Accuracy and Reliability in Outputs
Many businesses face challenges with AI models that produce inconsistent or inaccurate outputs, especially when applied to highly specific tasks. Fine-tuning AI models on relevant datasets tailored to your business can significantly improve both the accuracy and reliability of the model’s outputs.
Improve Output Accuracy and Reliability with Fine-Tuned Models
Pre-trained models, such as GPT-4o, often struggle with producing reliable results for specialized business tasks or industries where accuracy is critical. Fine-tuning these models ensures they are optimized to handle the unique challenges and requirements of your operations:
- Increased Output Accuracy: Fine-tuned models can process data with greater precision, ensuring that predictions, classifications, or generated outputs are aligned with the specific requirements of your business.
- More Reliable Results: By adapting the model to understand and predict business-specific patterns, fine-tuning reduces errors and ensures consistent performance over time.
- Task-Specific Optimization: Fine-tuning models for unique tasks such as document analysis, customer support, or personalized recommendations allows them to deliver highly accurate and relevant outputs.
How to Fine-Tune AI Models for Accuracy and Reliability
Here’s how you can fine-tune AI models to achieve more accurate and reliable outputs for your business:
- Identify Output Inconsistencies: Review current AI model outputs and identify areas where the model underperforms, providing inaccurate or inconsistent results.
- Collect Domain-Specific Data: Gather a dataset that reflects the nuances of your specific business needs (e.g., customer queries, or technical documents).
- Fine-Tune the Model on Relevant Data: Use FinetuneDB to fine-tune the model, teaching it to better understand and produce accurate results based on your specific requirements.
- Test and Validate the Model: Test the fine-tuned model across different scenarios to ensure its outputs are consistently accurate and reliable in your specific use cases.
- Deploy for Consistent Output: Once fine-tuned and validated, deploy the model in production to deliver reliable results across tasks, improving decision-making and efficiency.
Real Use Cases: Improving Accuracy and Reliability with Fine-Tuned Models
Businesses across various sectors have achieved significant improvements in accuracy and reliability by fine-tuning their AI models for specific, practical tasks:
- E-commerce Product Categorization: Online retailers fine-tune AI models to accurately classify products across diverse categories, improving search accuracy and reducing errors in product placement.
- Consistent Blog Writing Style: A travel blog fine-tunes AI models to generate blog posts that match their unique writing style and tone, ensuring all content feels cohesive and on-brand, while reducing the manual effort of writing articles from scratch.
- *Automated Feedback Categorization: An online retailer fine-tunes AI models to automatically sort customer feedback into categories such as shipping issues, product quality, and user experience.
Start Fine-Tuning AI Models for Accuracy and Reliability Today
Looking to improve the accuracy and reliability of your AI model outputs? Sign up at FinetuneDB, or contact us for a personalized demo and see how FinetuneDB can help your business fine-tune models to meet specific accuracy and reliability needs.